博客
Deep learning drives smart factories to achieve deep automation transformation
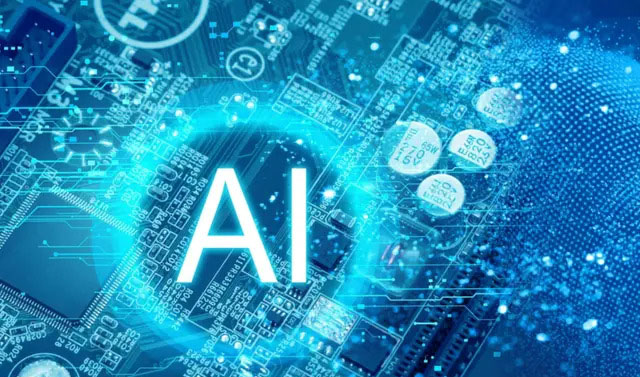
Introduction: The application of deep learning technology in smart factories has promoted the deep intelligent transformation of the manufacturing industry. Through the application of optimizing production processes, predictive maintenance, quality inspection, etc., deep learning has significantly improved production efficiency, reduced costs, and enhanced the flexibility and competitiveness of factories.
With the rapid development of information technology, intelligent manufacturing has become a key direction for the transformation and upgrading of the manufacturing industry. In this process, deep learning, as a core technology in the field of artificial intelligence, is gradually penetrating and profoundly changing the face of smart factories. This article will explore in depth how deep learning drives smart factories to achieve deep intelligent transformation, as well as the many benefits brought by this transformation.
1. Background and Challenges of Smart Factory
Traditional manufacturing faces challenges such as low production efficiency, serious waste of resources, and complex production processes. Especially when faced with small batch and multi-variety production needs, traditional methods are difficult to respond quickly to market changes. Therefore, the rise of smart manufacturing has become the key to solving these problems. Smart factories integrate technologies such as the Internet of Things (IoT), big data, and artificial intelligence to achieve intelligent and automated management of various links such as production, logistics, and quality inspection.
2. Potential and Application of Deep Learning
Deep learning has the ability to extract complex patterns from large amounts of data, making it very suitable for processing massive production data in smart factories. The following are several major applications of deep learning in smart factories:
Equipment health monitoring and predictive maintenance:
Deep learning can analyze equipment operation data in real time, such as vibration, temperature, current, etc., and detect signs of equipment failure in advance through predictive models, so as to perform preventive maintenance. This not only avoids equipment downtime and production interruptions, but also significantly reduces maintenance costs.
Production parameter optimization:
Deep learning can analyze the relationship between a large number of process parameters (such as temperature, pressure, speed, etc.) in the production process and product quality, and optimize the production parameter settings. This helps to improve product quality and production efficiency and achieve refined production.
Energy management:
By analyzing energy consumption data through deep learning models, the factory’s energy usage strategy can be optimized and energy waste can be reduced. This not only helps achieve energy conservation and consumption reduction goals, but also improves the economic benefits of the factory.
Intelligent quality inspection:
Using deep learning computer vision technology, real-time quality inspection of products on the production line can be performed. This technology can identify product defects, ensure the consistency and stability of product quality, and improve customer satisfaction.
Intelligent scheduling and dispatching:
Deep learning can optimize logistics scheduling and production scheduling inside and outside the factory. By predicting demand fluctuations, inventory levels, and production capacity, deep learning models can optimize resource allocation and improve supply chain efficiency.
3. Examples of Deep Intelligent Transformation
SAIC Volkswagen M uses more than 1,400 industrial robots and applies leading manufacturing technologies on a large scale to achieve intelligent and digital development of the entire manufacturing process. Through system interconnection and data value-driven, the MEB smart factory has gradually realized the intelligent transformation of manufacturing. Among them, deep learning technology plays an important role:
Production Service Bus (PSB) platform: This platform realizes device interconnection and data collection, providing a data foundation for the training of deep learning models.
Information management system: Integrates the central control systems of each workshop as well as the energy and environmental systems, intelligent logistics systems, quality management systems, etc. to form factory-level production data reports, providing strong support for the optimization of deep learning models.
4. Benefits of Deep Intelligent Transformation
The deep intelligent transformation of smart factories driven by deep learning brings many benefits:
Improve production efficiency: Deep learning significantly improves production efficiency by optimizing production processes and parameter settings.
Reduce costs: Predictive maintenance and energy consumption management reduce maintenance costs and energy waste.
Improve product quality: Intelligent quality detection ensures the consistency and stability of product quality.
Enhanced flexibility: Deep learning models can autonomously adjust production processes and parameters according to changes in production demand, achieving higher production flexibility and responsiveness.
5. Future Outlook
With the continuous advancement of technology and the expansion of application scenarios, the application of deep learning in smart factories will become more extensive and in-depth. Smart factories in the future will pay more attention to environmental protection and sustainable development, optimize energy use and waste disposal through deep learning, and achieve green manufacturing goals. At the same time, cross-factory collaborative optimization and full life cycle management will also